Advancing Urban Traffic Management with Deep Learning: A Holistic Approach to Optimizing Intelligent Infrastructure
Abstract
Urban traffic congestion remains a persistent and growing challenge in cities worldwide, driven by rapid urbanization and increasing vehicle ownership. Traditional traffic management systems often fall short in adapting to the dynamic nature of traffic flow and the rising demands of urban mobility. In recent years, deep learning has emerged as a promising solution, offering advanced methodologies for enhancing urban traffic management through intelligent infrastructure optimization. This paper provides a comprehensive examination of the integration of deep learning techniques into urban traffic systems, aiming to improve traffic flow, reduce congestion, and optimize infrastructure use. We explore various deep learning models, including Convolutional Neural Networks (CNNs), Long Short-Term Memory networks (LSTMs), and Reinforcement Learning (RL), and their applications in traffic prediction, incident detection, and adaptive traffic signal control. By leveraging real-time traffic data and historical patterns, these models facilitate more responsive and efficient traffic management solutions. Our analysis covers current methodologies, successful case studies, and the challenges faced in implementation, concluding with a discussion on future directions. We assert that incorporating deep learning into urban traffic management represents a significant leap towards smarter, more adaptive cities that can meet the evolving demands of urban mobility.
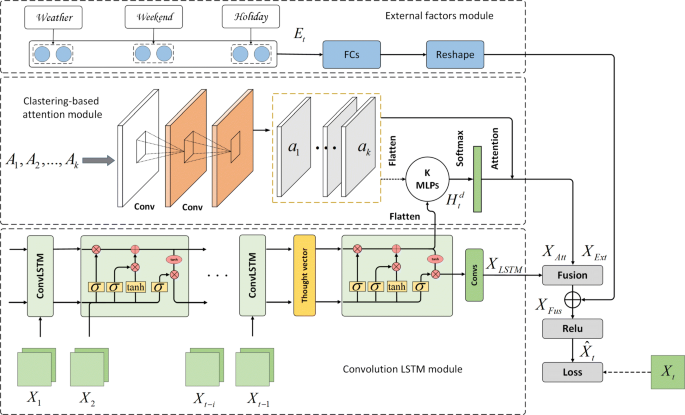
Downloads
Published
How to Cite
Issue
Section
License
Copyright (c) 2024 Tensorgate Journal of Sustainable Technology and Infrastructure for Developing Countries

This work is licensed under a Creative Commons Attribution-NonCommercial 4.0 International License.