Natural Language Processing Techniques for Clinical Text Analysis in Healthcare
Keywords:
Clinical Text Analysis, Natural Language Processing, NLP Techniques, Healthcare, Electronic Health Records, Information ExtractionAbstract
Natural Language Processing (NLP) techniques have emerged as valuable tools for clinical text analysis in healthcare. This study explores the various applications of NLP techniques in extracting meaningful information from unstructured clinical text data, such as electronic health records, medical literature, doctor's notes, and patient reports. The findings of this study highlight several commonly used NLP techniques and their significance in clinical text analysis.The first technique, tokenization, involves breaking down text into smaller units called tokens, enabling further analysis of the text. Named Entity Recognition (NER) is another technique employed to identify and classify named entities, including medical conditions, treatments, drugs, symptoms, and patient demographics. NER facilitates the extraction of relevant clinical information, aiding tasks such as coding diagnoses, medication reconciliation, and adverse event detection.Part-of-Speech Tagging (POS) assigns grammatical labels to words in a sentence, allowing for a better understanding of the syntactic structure of clinical text. This technique supports tasks like parsing, semantic analysis, and information extraction. Clinical coding, a vital process in healthcare, can be automated using NLP techniques, which streamline tasks such as assigning International Classification of Diseases (ICD) codes or Current Procedural Terminology (CPT) codes to clinical text, saving time and reducing errors.Information extraction techniques aim to identify specific pieces of information from clinical text, including drug dosages, laboratory values, treatment plans, and patient outcomes. This extracted information finds utility in decision support systems, clinical research, and quality improvement initiatives. Text classification categorizes clinical text into predefined categories, facilitating organization and retrieval of clinical information. Sentiment analysis, on the other hand, determines the emotional tone expressed in clinical text, aiding in understanding patient satisfaction, identifying adverse events, and assessing sentiment towards drugs or treatments.Language modeling techniques, such as word embeddings and contextual embeddings, capture semantic relationships in clinical text. These models enable tasks like semantic similarity, text generation, and language understanding. NLP techniques can be employed to develop clinical question-answering systems, interpreting and answering medical questions based on clinical text, literature, guidelines, or medical ontologies.The integration of NLP techniques into clinical decision support systems enhances healthcare delivery by extracting relevant information from clinical text and providing context-aware recommendations, alerts, or guidelines to healthcare providers at the point of care. These findings emphasize the versatility of NLP techniques and their potential to improve healthcare delivery, research, and patient outcomes.As the field of NLP in healthcare continues to evolve, researchers and practitioners are continuously developing new techniques and applications. The rapid progress in this area holds promise for the future of healthcare, offering opportunities to advance healthcare practices, improve research methodologies, and enhance patient outcomes.
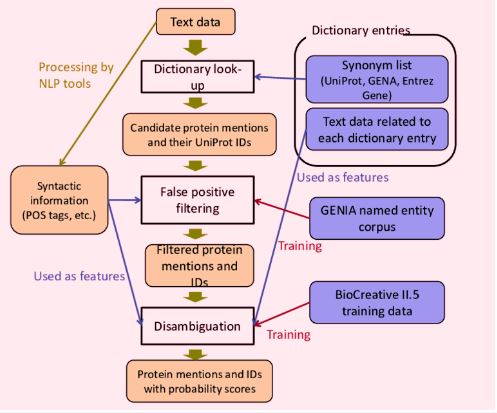