Hybrid Adaptive Fault Detection and Diagnosis System for Cleaning Robots
Keywords:
Fault Detection and Diagnosis, Cleaning Robots, Hybrid System, Machine Learning, Digital Twin, Adaptive LearningAbstract
Background: Fault Detection and Diagnosis (FDD) in robotic systems, particularly cleaning robots, is required for maintaining operational efficiency and reliability. Traditional FDD approaches, categorized as data-driven, model-based, and knowledge-based, have their individual strengths and limitations.
Objective: This research proposes a novel Hybrid Adaptive FDD System, integrating the three traditional approaches to create a robust, efficient, and adaptive fault detection system for cleaning robots.
Methods: The proposed system combines i) a data-driven layer utilizing machine learning algorithms, ii) a model-based layer employing a digital twin for performance comparison, and iii) a knowledge-based layer with a comprehensive database of common cleaning robot faults. An adaptive learning component is integral to the system, facilitating continuous learning and updating of the FDD algorithms and knowledge base.
Experimental Setup: The system was implemented and tested on a fleet of cleaning robots in a controlled environment. The robots were equipped with various sensors to collect real-time operational data, which were then processed and analyzed by the proposed FDD system.
Results: Experimental results demonstrate that the Hybrid Adaptive FDD System is capable of reliably detecting the presence of faults in cleaning robots. The system showed high accuracy in identifying anomalies in operational patterns, mechanical or software faults, and matched observed anomalies with known fault patterns effectively.
Conclusion: The integration of data-driven, model-based, and knowledge-based approaches in a single FDD system ensures its applicability and effectiveness in dynamic operational environments. This research contributes to the field of robotic maintenance by providing an adaptive, and efficient solution for fault detection and diagnosis in cleaning robots.
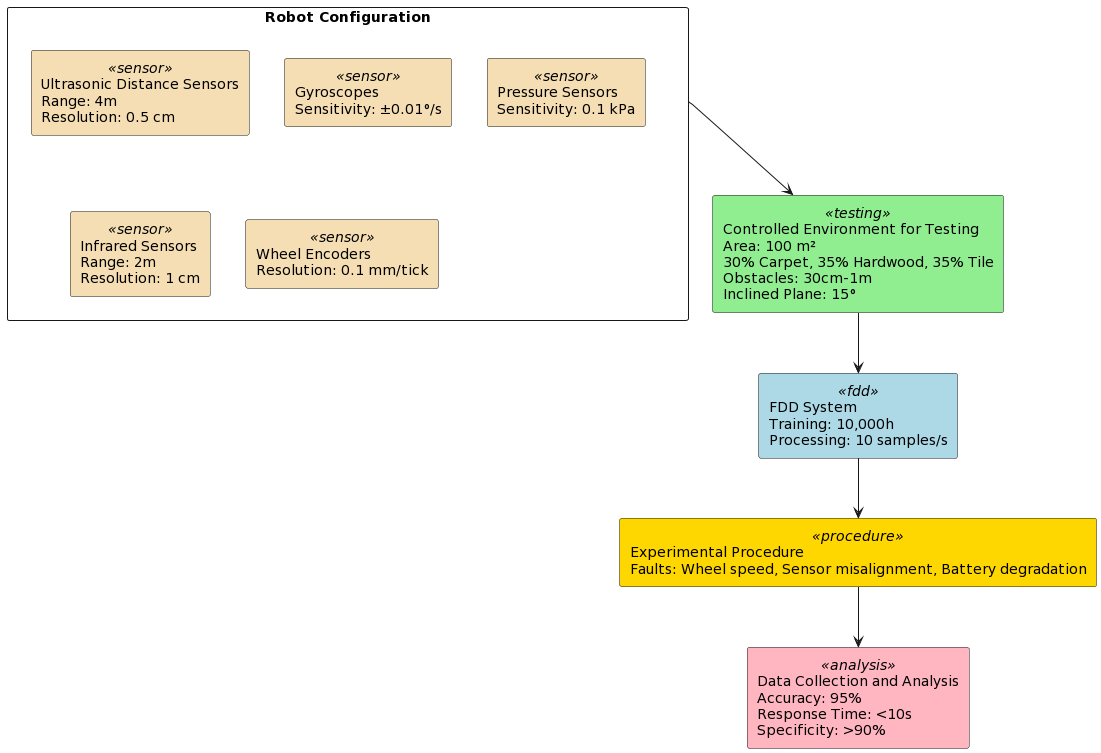
Downloads
Published
How to Cite
Issue
Section
License
Copyright (c) 2024 International Journal of Intelligent Automation and Computing

This work is licensed under a Creative Commons Attribution-NonCommercial 4.0 International License.